Media Monitoring with Big Data: Opportunities and Challenges
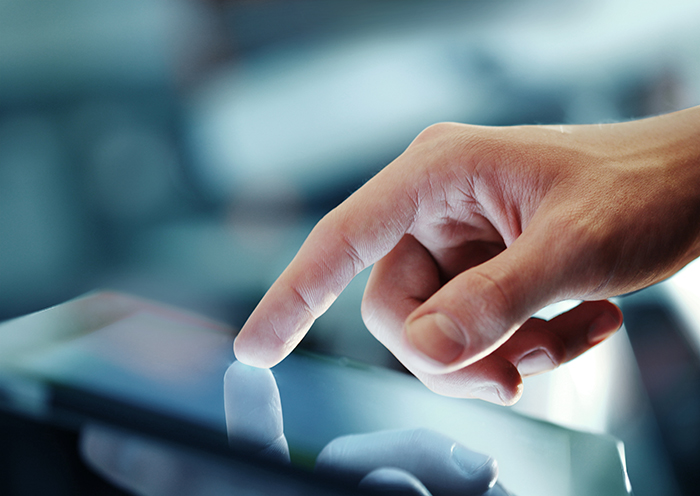
What would marketing strategists do if every second of all broadcast television, broadcast radio, and social media posts were available in real time for analysis? How would advertisers react if the transcripts of all the TV and radio broadcast information were available in addition to video and audio for every second anywhere in the nation in real time? Big Data analytics provides a means to digest this copious amount of information and to make ad buying decisions on the fly.
Big Data analytics means not only processing very large amounts of data but also attempting to coherently connect the unstructured nature of the data. For example, the data could be a combination of radio and TV transmissions converted into speech by a speech coder, closed caption text of TV programs, Twitter and Facebook posts, financial data and spreadsheets, and news articles. Another advantage of Big Data analytics is that the system is self-learning; once the initial analytical model has been created, additional new data and information can be easily integrated into the knowledge base. Big Data analytics has been creating a buzz in the industry for a number of years, and many companies are already offering tools and services to reap the benefits.
An example of a company that archives broadcast content for media monitoring is Critical Mention. Critical Mention captures and indexes 40 hours of broadcast content every 60 seconds from more than 2,000 unique broadcast sources. The users thus have access to a database of more than 16 million searchable segments, all available in broadcast quality and near-real time. The users can search TV/radio/online news, watch video, edit and share coverage, receive real-time alerts, and create reports. The system also offers analytic tools to gain insight into the data, to benchmark against competitors, to analyze viewer sentiment, and to visualize density of coverage on maps. The following picture is an example of the analysis tool output.
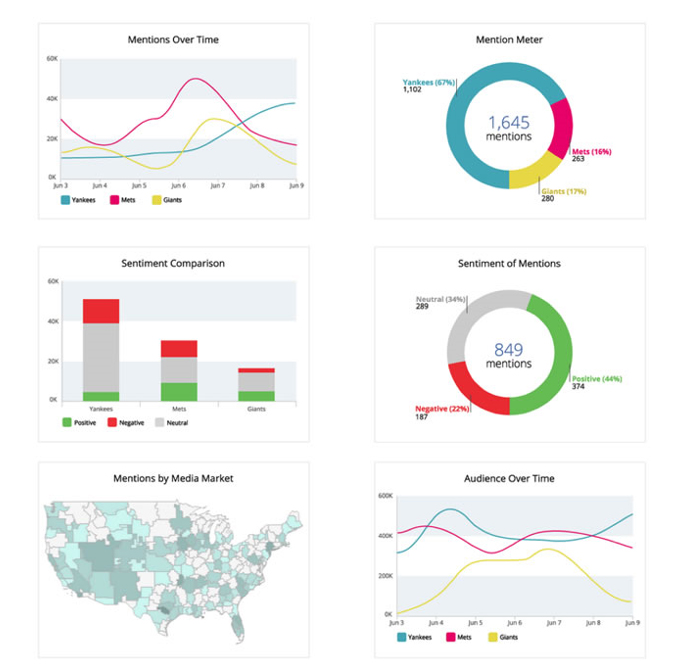
Figure: Critical Mention Analytics
Figure: Critical Mention Analytics
Integration of Big Data analytics with archived media information would open up many new possibilities in the future. For example, the single string-based search provided by Critical Mention can be augmented to perform searches based on different combinations of groups of words. Grouping of related words and inclusion/exclusion combinations of many different groups are known as dictionaries and rules in Big Data jargon, and these well known techniques are used to isolate relevant information and validate hypotheses. The natural language processing (NLP) capabilities of big data can also help make inference about the context in which the key words are used, making the results more reliable and relevant. Yet one can envision that image processing and pattern matching algorithms could be used to detect and quantify product placement in broadcast video. For example, the Coke logo can be identified in time-stamped video frames across all the broadcasters of all the DMAs in real time. These capabilities are computationally intensive, but the algorithms are fairly well understood. Cloud services, which offer scalable computational resources, can make this kind of analysis available in the future.
Recognizing some of these potential business opportunities, broadcasters and consumer electronics manufacturers are developing a next-generation television system with dynamic ad insertion and targeted advertising capabilities. If ad buyers can identify a need – in real-time if they wish – to maintain their brands’ share of voice (SOV) in certain geographically segmented markets, broadcasters will have the technical means to deliver the ads to the intended audience in the future. As a consequence, programmatic advertising and ad exchange might become more dynamic in terms of balancing demand and supply.
This kind of individualized targeting might also raise challenges to traditional advertising models in the broadcast industry. With access to all the real-time and personalized information of the audience, an ad buyer will have the option to use different means to reach the audience, even when the audience is consuming broadcast content. For example, armed with the knowledge of a consumer’s state of mind at a certain location at a certain instant, the ad buyer might reach the targeted customer through a smartphone, thus totally bypassing the broadcasters. Proliferation of the Internet of Things (IoT) will provide more outlets for targeted advertising, and the situation might become even more challenging for the traditional broadcasting model.
Media monitoring along with Big Data analytics has tremendous potential for targeted and contextual advertising. The advertising industry is changing rapidly, revealing both opportunities and challenges for broadcasters. Broadcasters will need to be vigilant of this fundamental paradigm shift in advertising and adapt to new market challenges.